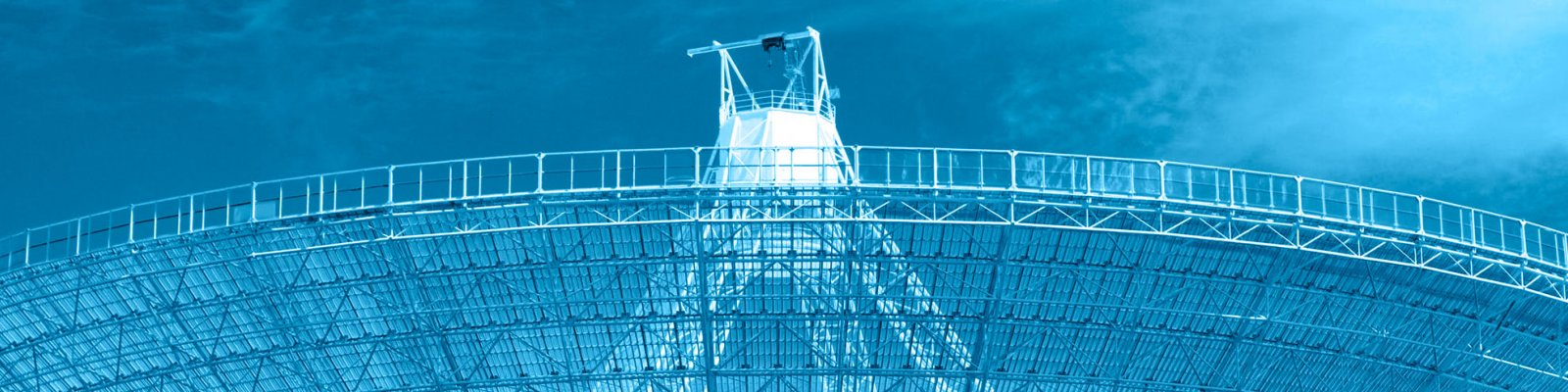
The primary source of climate model data presented in our maps, charts and tables is the Pacific Climate Impacts Consortium (PCIC). [1] PCIC has provided us with downscaled projections of daily temperature and precipitation data from 24 climate models using two carbon emission scenarios. Here, we provide an overview of this dataset, a brief description of what downscaling means, and a technical overview of the specific type of downscaling used.
About these data
We use PCIC’s statistically downscaled data (Bias Correction with Constructed Analogues and Quantile mapping, Version 2; BCCAQv2) derived from 24 CMIP5 global climate models (the complete list of models can be found below), for two emissions scenarios (RCP4.5 and RCP8.5). We call the RCP4.5 and RCP8.5 the “Low Carbon” and “High Carbon” scenarios, respectively.
Read more: Full list of climate models included in the ensemble
Model Name | Original Resolution (lat/lon) | Institution |
---|---|---|
bcc-csm1-1 | 2.81° x 2.81° | Beijing Climate Center, China Meteorological Administration |
bcc-csm1-1-m | 1.12° x 1.12° | Beijing Climate Center, China Meteorological Administration |
NorESM1-M | 2.50° x 1.87° | Norwegian Climate Centre |
NorESM1-ME | 2.50° x 1.87° | Norwegian Climate Centre |
MRI-CGCM3 | 1.12° x 1.12° | Meteorological Research Institute |
MPI-ESM-LR | 1.87° x 1.87° | Max Planck Institute for Meteorology (MPI-M) |
MPI-ESM-MR | 1.87° x 1.87° | Max Planck Institute for Meteorology (MPI-M) |
MIROC5 | 1.40° x 1.40° | Atmosphere and Ocean Research Institute (The University of Tokyo), National Institute for Environmental Studies, and Japan Agency for Marine-Earth Science and Technology |
MIROC-ESM | 2.81° x 2.81° |
Japan Agency for Marine-Earth Science and Technology, Atmosphere and Ocean Research Institute (The University of Tokyo), and National Institute for Environmental Studies |
MIROC-ESM-CHEM | 2.81° x 2.81° |
Japan Agency for Marine-Earth Science and Technology, Atmosphere and Ocean Research Institute (The University of Tokyo), and National Institute for Environmental Studies |
HadGEM2-ES | 1.87° x 1.24° |
Met Office Hadley Centre (additional HadGEM2-ES realizations contributed by Instituto Nacional de Pesquisas Espaciais) |
HadGEM2-AO | 1.87° x 1.24° |
Met Office Hadley Centre |
GFDL-ESM2M | 2.50° x 2.00° |
Geophysical Fluid Dynamics Laboratory |
GFDL-ESM2G | 2.50° x 2.00° |
Geophysical Fluid Dynamics Laboratory |
GFDL-CM3 | 2.50° x 2.00° |
Geophysical Fluid Dynamics Laboratory |
FGOALS-g2 | 2.81° x 3.00° |
LASG, Institute of Atmospheric Physics, Chinese Academy of Sciences; and CESS, Tsinghua University |
CanESM2 | 2.81° x 2.81° |
Canadian Centre for Climate Modelling and Analysis |
CSIRO-Mk3-6-0 | 1.87° x 1.87° |
Commonwealth Scientific and Industrial Research Organisation in collaboration with the Queensland Climate Change Centre of Excellence |
CNRM-CM5 | 1.40° x 1.40° |
Centre National de Recherches Meteorologiques / Centre Europeen de Recherche et Formation Avancees en Calcul Scientifique |
CCSM4 | 1.25° x 0.94° |
National Center for Atmospheric Research |
BNU-ESM | 2.81° x 2.18° |
College of Global Change and Earth System Science, Beijing Normal University |
IPSL-CM5A-MR | 2.50° x 1.25° |
Institut Pierre-Simon Laplace |
IPSL-CM5A-LR | 3.75° x 1.87° |
Institut Pierre-Simon Laplace |
CESM1-CAM5 | 1.40° x 1.40° |
National Science Foundation, Department of Energy, National Center for Atmospheric Research |
Table adapted from Li et al. (2018) [5]
For each model/scenario the PCIC dataset provides daily temperatures (maximum and minimum) and total precipitation at a 10 km x 10 km resolution for all of Canada, for the period 1950-2100. Because some of the models did not have data beyond 2095, we used this year as our cutoff for future time series.
For each model, the simulations for 1950-2005 are the same for both emissions scenarios (RCP4.5 and RCP8.5). The divergent emissions scenarios were used by the models starting in 2006. That is, starting in 2006 the model outputs for the two RCPs begin to differ.
Climate projections are provided for two future 30-year periods (2021-2050 and 2051-2080) and a baseline period (1976-2005). Unless otherwise stated, the maps and data we present are the averages of the 24 models.
Please visit our page “Important Data Notes and Limitations” to learn more about these data.
What does downscaling mean?
As the name would suggest, Global Climate Models (GCMs) (also known as General Circulation Models) are built to evaluate global-scale climate conditions. However, it is important to note that all GCM projections can suffer from so-called ‘systematic biases’ when they are used to analyze local-scale climates. Moreover, GCM datasets are generally calculated at fairly coarse spatial resolutions (>1° lat/lon, or more than 100 km x 100 km in southern Canada) that further impacts the value of the original GCM data for studying local climate changes. Therefore, to improve the utility of GCM projections for local-scale analyses of climate change, scientists employ various types of systematic bias-correction and spatial downscaling techniques.
Generally, bias correction and downscaling are done at the same time. Bias correction/downscaling comes in two flavours: dynamic and statistical. The purpose of both types is to remove systematic bias within the data, as much as possible, and to convert the coarse-resolution GCM data into higher-resolution data (with data points closer together than in the original model output).
The scientific literature contains several reviews of downscaling techniques. For example, Bürger et al. (2012) and Bürger et al. (2013) compared numerous types of statistical downscaling to evaluate how well they reproduced both observed and projected future climatic extremes. [2] [3] Pierce et al. (2013) compared RCM, GCM and two different statistical downscaling methodologies to compare projections of extreme precipitation events in California. [4] More recently, Li et al. (2018) evaluates several climate change indices related to agriculture and adaptation planning using statistically downscaled climate data. [5]
What method of downscaling did we use?
The climate model data presented in the Atlas has been statistically downscaled and bias corrected using a method called Bias-Correction/Constructed Analogues with Quantile mapping reordering, Version 2 (BCCAQv2); the work was done by the Pacific Climate Impacts Consortium (PCIC). [1] This method has been extensively tested by Murdock et al. (2014) and found to outperform many other statistical downscaling methodologies. [6]
BCCAQ is a hybrid method (Maurer et al. 2010). [7] First, a method called Bias Correction/Constructed Analogues (BCCA) is used to rescale projections to match observations using a calibration period of 1950-2010. While BCCA has been found to outperform several statistical downscaling and bias correcting methods, it has also been found to interfere with or deteriorate the underlying trend computed by the GCM in some cases (Cannon et al. 2015). [8] Therefore a second method called quantile mapping is employed to preserve the original GCM trends in the downscaled daily data (Gudmundsson et al. 2012). [9] Li et al. (2018) verified that this version of BCCAQ data (Version 2) describes historical climate observation very well. [5] While the BCCAQ method performs well compared to many other statistical downscaling methods, Murdock et al. (2014) do caution that the method is prone to producing “alarmingly large relative increases” in precipitation for some locations. [6]
PCIC has computed and made available BCCAQv2 downscaled data for 27 GCMs included in the CMIP5 project. Here, however, we elected to use the same 24 models used by Li et al. (2018). [5] In addition, three representative concentration pathways (RCPs) are available: RCP8.5 (hereafter referred to as the ‘High Carbon’ scenario), RCP4.5 (‘Low Carbon’) and RCP2.6 (‘Very Low Carbon’). Here, we use only the RCP8.5 and RCP4.5 emissions scenarios in our analysis. We elected to not use RCP2.6 data at this time, to simplify the mapping project and because it seems very unlikely to be a future reality. Data pertaining to RCP6.0 was not available from PCIC.
Observed Data
For comparative purposes, historical data for the period 1950-2013 are included in all time-series graphs. By providing both historical and modelled data over this period, it is possible for users to visually judge how well model projections match historical data over this 64-year period.
The source of the daily historical data used in the atlas is Natural Resources Canada (NRCan; McKenney et al., 2011). [10] This dataset is comprised of daily maximum and minimum air temperature and daily precipitation totals for the same 10 km by 10 km grid used in the downscaled GCM datasets produced by PCIC.
Please visit our page “Important Data Notes and Limitations” to learn more about these data.
Methodology
The fine-grid model data arrays produced by PCIC are too large to process directly using GIS software, and the computational power and time needed to process the high resolution data from 24 models was found to be very high. Consequently, we developed software using Python to unpack the model data, compute summary statistics, and produce smaller output files that could be visualized and turned into map tiles using QGIS.
Therefore, the maps in the Atlas do not contain the original 10 km by 10 km data. Areal averages are provided for two map scales used by the National Topographic Service (NTS) (1:250,000 and 1:50,000), and also for Canada’s Provinces and Territories. The NTS grids were selected because they are available for all of Canada, have a spatially and climatically appropriate size, and are helpfully named according to local landforms or major population centres, making them recognizable and useful to web users.
In addition to the spatially averaged data, nearest-neighbour analysis was used to compute point-value data for approximately 500 towns and cities across Canada. Again, this was done for the benefit of the web user; having data summaries made available for cities and towns makes these model data accessible and relevant for a wide sector the Canadian population. Indeed, one of the major objectives of the Atlas is to show Canadians how climate change is expected to change their local climates.
Please visit our page “Important Data Notes and Limitations” to learn more about these data.
Sources of Non-Climate Map Data
Highways and Winter Roads Map Layers. Statistics Canada, 2015. National Road Network.
Indigenous Territories Map Layer. Native Land, 2018.
Watershed Map Layer. Water Survey of Canada (Environment Canada). Year of original publication unknown. Made available by ESRICanadaEducation through ESRI’s ArcOnline service.
National Topographic System (NTS) Map Layers. Government of Canada National Topographic System, 2017. 1:250,000 and 1:50,000 map scales. Made available by the Government of Canada through ESRI’s ArcOnline service.
Legal Disclaimer
The information disseminated by the Prairie Climate Centre including but not restricted to maps, tables, statistics and interpretations is provided as a public service. It is provided without any warranty or representation, expressed or implied, as to its accuracy or completeness. Any reliance you place upon the information contained here is your sole responsibility and strictly at your own risk. In no event will the Prairie Climate Centre be liable for any loss or damage whatsoever, including without limitation, indirect or consequential loss or damage, arising from reliance upon the data or derived information.
References
- Pacific Climate Impacts Consortium, University of Victoria, (Jan. 2014). Statistically Downscaled Climate Scenarios.
- Bürger, G., Murdock, T., Werner, A., Sobie, S., 2012. Downscaling extremes-an intercomparison of multiple statistical methods for present climate. Journal of Climate, 25: 4366-4388.
- Bürger, G., Sobie, S., Cannon, A., Werner, A., and Murdock, T., 2013. Downscaling extremes: an intercomparison of multiple methods for future climate. Journal of Climate, 26: 3429-3449.
- Pierce, D., Cayan, D., Das, T., Maurer, E., Miller, N., Bao, Y., Kanamitsu, M., Yoshimura, K., Snyder, M., Sloan, L., Franco, G., Tyree, M., 2013. The key role of heavy precipitation events in climate model disagreements of future annual precipitation changes in California. Journal of Climate, 26: 5879-5896.
- Li, G., Zhang, X., Cannon, A., Murdock, T., Sobie, S., Zwiers, F., Anderson, K., and Qian, B. (2018). Indices of Canada’s future climate for general and agricultural adaptation applications. Climatic Change (2018) 148:249–263 https://doi.org/10.1007/s10584-018-2199-x
- Murdock, T., Sobie, S., Hiebert, J., 2014. Statistical downscaling of future climate projections for North America: report on contract no: KM040-131148/A. Available online: https://www.pacificclimate.org/sites/default/files/publications/PCIC_EC_downscaling_report_2014.pdf
- Maurer, E., Hidalgo, H., Das, T., Dettinger, M., Cayan, D., 2010. The utility of daily large-scale climate data in the assessment of climate change impacts on daily streamflow in California. Hydrology and Earth System Science, 14: 1125-1138.
- Cannon, A., Sobie, S., Murdock, T., 2015. Bias correction of GCM precipitation by quantile mapping: how well do methods preserve changes in quantiles and extremes? Journal of Climate, 28: 6938-6959.
- Gudmundsson, L., Bremnes, J., Haugen, J., Engen-Skaugen, T., 2012. Technical note: downscaling RCM precipitation to the station scale using statistical transformations - a comparison of methods. Hydrology and Earth System Science, 16: 3383-3390.
- McKenney, D. W., Hutchinson, M.F., Papadopol, P., Lawrence, K., Pedlar, J., Campbell, K., Milewska, E., Hopkinson, R., Price, D., Owen, T. (2011). "Customized spatial climate models for North America." Bulletin of American Meteorological Society December: 1612-1622. https://journals.ametsoc.org/doi/pdf/10.1175/2011BAMS3132.1