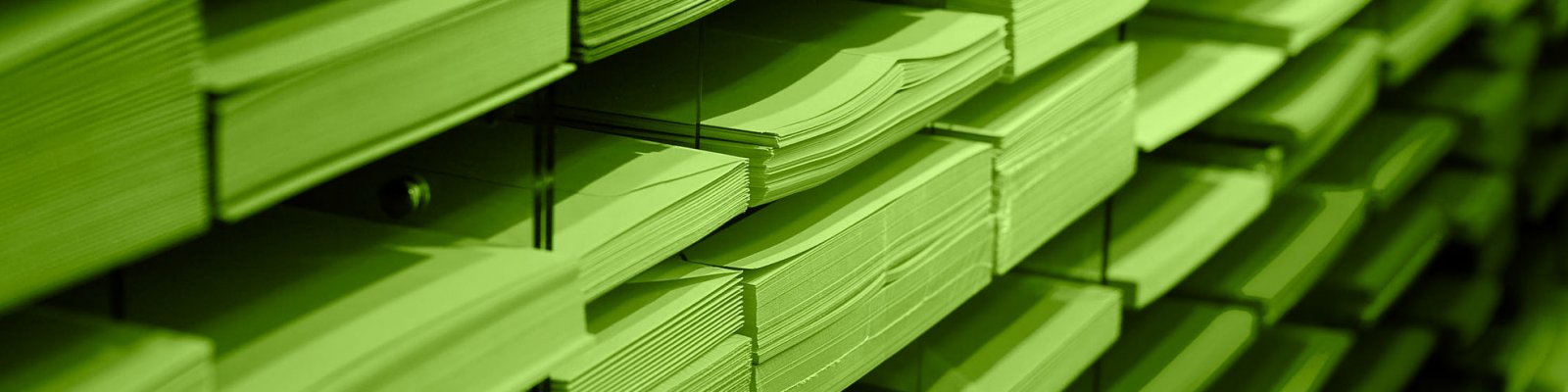
The following caveats should be reviewed by anyone intending to use data from the Climate Atlas for decision-making. These caveats are especially pertinent for engineers, planners and other professionals looking for climate data to inform their work.
Downscaling Method
The climate model data used in this version of the Climate Atlas was statistically downscaled by the Pacific Climate Impacts Consortium (PCIC) using Version 2 of the Bias Correction with Constructed Analogues and Quantile mapping (BCCAQv2) method. The BCCAQv2 method is an improvement upon the Bias Correction Spatial Disaggregation (BCSD) method used in the original previous version of the Atlas.
The older BCSD algorithm is a relatively simple downscaling method. It used an historical climate dataset produced by Natural Resources Canada (NRCan) to generate simulated daily variability from monthly climate model data. Daily values were sampled randomly from historical months in the NRCan dataset and then rescaled so that the monthly means matched the original climate model projections. Importantly, this meant that the BCSD downscaled daily data reflected changes in the monthly means, but not changes in daily variability within the original model output.
The BCCAQv2 method uses the same NRCan ‘training’ dataset used to create the BCSD data. Therefore, not surprisingly, the two downscaling methods compare very well when one compares climate changes across years or seasons. However, unlike BCSD, the new BCCAQv2 method used in this version of the Atlas retains the climate models’ original daily time steps, meaning changes in daily variability in the downscaled data now much better reflect the original global model output.
In this version of the Atlas, we present data from 24 climate models. This is a substantial increase from the previous version of the Atlas, which used a 12-model ensemble. Generally speaking, this increase in the number of models increases the range of the projections.
The full BCCAQv2 dataset made available by PCIC actually contains 27 climate models. Moreover, multiple runs from the same model were also available. For consistency, we use the same 24 models provided by Environment and Climate Change Canada (ECCC) via the online climate data viewer. (ECCC’s website was constructed at a time when only 24 models were ready for visualization.)
Ensembles
Unless otherwise indicated, the data presented in the Atlas depicts the average, or ensemble mean, of the statistically downscaled data from the 24 climate models. It is standard practice in climatological studies to use data not from one model but a range of models, to represent the uncertainty associated with the modeling process. For the sake of simplicity, we chose to use the mean of the ensemble, rather than the median values, as is done in some studies. Importantly, for some of our depictions, the mean value is accompanied by the 90th and 10th percentile values from the 24 models, to indicate the range of projections across the models.
Seasons
We define the four seasons in the standard climatological manner: Winter = December / January / February; Spring = March / April / May; Summer = June / July / August; and Fall = September / October / November. For almost all parts of Canada, one or more of these sets of months will fail to capture the true start and end dates of the seasons. Furthermore, choosing specific months to define seasons does not take into account the inevitable shifts in climate that will come to define our seasons in the future.
Spatial Resolution and Interpolation
The spatial downscaling process used by PCIC relies upon the Natural Resources Canada (NRCan) gridded dataset of historical daily meteorological observations to represent how temperature and precipitation varies in space. Although the gridded data is generally of high quality, the quality is spatially inhomogenous. In particular, the quality of the NRCan data is reduced in this parts of Canada where there are i) temporal gaps in the weather station data; ii) large geographic distances between weather stations; iii) mountainous terrain; and/or iv) large contrasts in microclimate in a region. In short, the climate model data we use is most prone to interpolation error over mountainous regions, and in areas with few long term weather stations, such as northern Canada.
Nearest Neighbour Analysis
Climate change values for municipalities (cities and towns) were computed by finding the nearest neighbouring grid point. That is, downscaled data from single grid points representing a 10 km by 10 km area were used to represent the climate of cities and towns, even though those places may be larger than this area. This choice was made for the sake of computational simplicity.
Areal Averaging
The Atlas allows users to explore climate change values across all of Canada, including remote and rural areas. However, due to computational and internet server limitations, we cannot provide all the original model data at its native 10 km resolution. For this version of the Atlas, we have opted to areally average the data at three scales:
- National Topographic Service (NTS) of Canada 1:250,000 topographic map areas;
- NTS of Canada 1:50,000 topographic map areas;
- Provinces and Territories.
Only data points completely contained within the spatial domains of these regions were included in their areal averages.
Non-Ocean Data
It is important to note that the gridded model data used to create the Atlas was available for virtually all areas of Canada including lakes, such as Lake Winnipeg, but not including all of the Great Lakes, which extend into the United States). No data was available for ocean surfaces. Many of the NTS grid squares along Canada’s coasts contain large areas of ocean, sometimes with only a tiny fraction of the area being land. In these cases, the areal-averaged value for these coastal grid squares only represent conditions over the terrestrial portion of the grid. The number of data points included in any area is indicated in the sidebar on the atlas map.
Confidence and Impacts
It is impossible to state with certainty that a specific projected climate change will occur. In fact, any expectation of absolute confidence from climate models is an ill-fated quest; there will always be uncertainty associated with what the models say about how the climate is expected to change. Furthermore, it is impossible to say with any certainty that the climate change that does occur will have a particular impact. However, the level of confidence one might have in possible impacts will vary from case to case, depending on the kind of climate change associated with the impact, and the nature of the relationship between the change and the impact of that change.
For example, it is one thing to compute the number of +30 °C days projected for some time in the future, but quite another to state how an increase in +30 °C days will likely impact human health, forest fire frequency, or thunderstorm intensity, and another still to calculate the cumulative impact these impacts have on the economy.
We might have much higher confidence in stating that an increase in the number of hot days will likely increase the number of heat strokes in urban environments (unless adaptation occurs), but lower confidence that the increase in these hot days will increase the severity of thunderstorms (which require specific kinds of meteorological conditions). That is, there is a quite direct relationship between heat-related illnesses and high outdoor temperatures, whereas thunderstorm development depends on many environmental factors, with temperature being just one. Similarly, we are likely to have much lower confidence in stating that these impacts will negatively affect the nation’s economy. Our level of confidence decreases as the system becomes more complex and more variables come into play.
Where we have lower confidence in our ability to make meaning from the data, we surveyed the most up-to-date peer-reviewed literature and connected with experts from across Canada to better inform our work. However, we will never be able to predict future impacts with absolute certainty. In spite of this, we strongly believe that society needs to move forward to address the risks of climate change in the face of uncertainty. Indeed, this is one of the goals of our work: to initiate discussions about how the climate is likely to change, what the impacts of these changes may be, and how we should respond.
Specific Climate Variables and Indices
Precipitation
Precipitation is much more difficult to model than temperature. Consequently, we have relatively low confidence in the point-specific, time-specific precipitation projections. However, we have higher confidence in the projections when they are aggregated to produce longer-term averages of monthly, seasonal and annual precipitation totals.
Note that the range of projected precipitation values across the models used to create our ensemble values is relatively high compared to the range of values in the temperature projections; this is typical of all climate model studies.
Heavy Precipitation Days
The Climate Atlas shows relatively little change in the number of heavy precipitation days (HPD) in the future; however, there is much uncertainty regarding how well climate models capture these intense and often localized events (such as thunderstorms).
Nevertheless, climate scientists are quite confident that the number of heavy precipitation events — and especially rainfall events – will more frequent and more intense in the future, as the atmosphere becomes more energetic and moist. Indeed, there is an abundance of evidence that rainstorms in many parts of the world, including North America, are now more frequent and intense.
Consequently, we have low confidence in the HPD values. More specifically, it is likely that the projections underestimate the frequency and intensity of heavy precipitation events. We have higher confidence in the longer-term averages of monthly, seasonal and annual precipitation totals represented in the Atlas.
Mean Temperature
In meteorology, the daily mean temperature is usually calculated as the average of all temperature measurements made in a day (e.g. the average of 24 individual hourly measurements). It is common in climatological research to calculate daily mean temperature as the average of the daily maximum and minimum temperatures. On daily time-scales, the two methods for computing the daily mean can yield slightly different results.
Because we only had access to modelled daily maximum and daily minimum temperatures, we define the daily mean temperature as the average of the daily maximum and minimum temperature. All of the mean temperature values in the Atlas (including historical observed values) have been calculated using the same method, making them internally consistent and comparable.
Date of First Fall Frost, Date of Last Spring Frost, Frost-Free Season
The temperatures we use to compute the length of the frost-free season are based on standard weather station observations, which are captured 1.2 m above the ground. Minimum temperatures at or near ground level, where plants emerge and grow, can vary quite a bit from this standard surface air temperature. Thus, the standard method of identifying the presence or absence of frost is an approximation. As the minimum temperatures measured at ground level are often colder than those at 1.2 m above the ground, the estimated length of the frost-free season presented here is likely slightly longer than the actual length of the ground-level frost-free season.